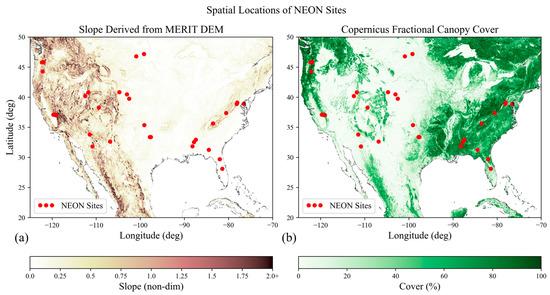
A new paper, “Modeling Uncertainty of GEDI Clear-Sky Terrain Height Retrievals Using a Mixture Density Network”, was recently published in the Special Issue of Remote Sensing and Ecosystem Modeling for Nature-Based Solutions by The University of Texas Center for Space Research Ph.D student, Jonathan Sipps, about innovative solutions for remote sensing analytics using machine learning to model uncertainties of space-based laser altimetry at the global scale.
Abstract: Early spaceborne laser altimetry mission development starts in pre-phase A design, where diverse ideas are evaluated against mission science requirements. A key challenge is predicting realistic instrument performance through forward modeling at an arbitrary spatial scale. Analytical evaluations compromise accuracy for speed, while radiative transfer modeling is not applicable at the global scale due to computational expense. Instead of predicting the arbitrary properties of a lidar measurement, we develop a baseline theory to predict only the distribution of uncertainty, specifically for the terrain elevation retrieval based on terrain slope and fractional canopy cover features through a deep neural network Gaussian mixture model, also known as a mixture density network (MDN). Training data were created from differencing geocorrected Global Ecosystem Dynamics Investigation (GEDI) L2B elevation measurements with 32 independent reference lidar datasets in the contiguous U.S. from the National Ecological Observatory Network. We trained the MDN and selected hyperparameters based on the regional distribution predictive capability. On average, the relative error of the equivalent standard deviation of the predicted regional distributions was 15.9%, with some anomalies in accuracy due to generalization and insufficient feature diversity and correlation. As an application, we predict the percent of elevation residuals of a GEDI-like lidar within a given mission threshold from 60°S to 78.25°N, which correlates to a qualitative understanding of prediction accuracy and instrument performance.
Source: https://www.mdpi.com/2072-4292/15/23/5594